Can contextualized ECG data collected (via Mobile Health Technology) under free-living conditions help improve ambulatory arrhythmia monitoring and diagnosis?
Find the PhD here
Background
The World Health Organization Health Statistics report listed cardiovascular diseases (CVD) as the number one cause of global deaths [1]. In 2016 alone, nearly 17.9 million people died because of CVD, and roughly 85% of these deaths were attributed to heart attack and stroke [1]. Cardiac arrhythmias comprise a large class of CVD and are associated with an increased risk of heart attack and stroke. In addition, arrhythmia like AF has huge healthcare costs (nearly $26 billion in the US and roughly €73–95 million annually in Denmark) [2-3].
Electrocardiogram (ECG) analysis is a cost-effective and non-invasive means for detecting heart arrhythmia. However, due to their sporadic and often asymptomatic nature, it is difficult to diagnose arrhythmias (in the early stages) during routine hospital ECG examinations. They require more extended continuous ECG monitoring in patients' free-living conditions. Even though longitudinal monitoring in a patient's free-living conditions can help in the early diagnosis of arrhythmias, it suffers from a lack of sustained patient engagement, poor signal quality, and recall bias in the patient's self-reported symptoms/activities diary, and a high false-positive rate (FPR) in computer-aided automatic arrhythmia detection algorithms. The high FPR in longitudinal screening increases the workload of clinicians (as it requires manual review) and could also lead to over-diagnosis and patient anxiety [4-5].
Objectives
This project investigates the role of context-awareness obtained via mobile and wearable devices to improve ambulatory arrhythmia analysis and reduce the FPR under free-living conditions. In addition, we also address the issues of recall bias in the patient-reported symptoms diary and events and sustained patient engagement in longitudinal arrhythmia screening.
First, we identified the context information relevant for improving ambulatory arrhythmia screening under free-living conditions. After that, mCardia – a context-aware ECG collection system was designed and its clinical feasibility and usability was evaluated for longitudinal arrhythmia screening under free-living ambulatory conditions. The mCardia system design addressed the problem of recall bias in patient-reported symptoms/event diary and sustained patient engagement in longitudinal screening. Two clinical case studies from the collected contextualized data demonstrated the usefulness of contextual data in improving the manual analysis of ECG.
For improving the automated AF detection algorithm under free-living conditions, we first investigated the influence of the patient's ambulatory context on FPR in a state-of-the-art AF detection algorithm. We found that three specific contexts: changes in activity, changes in body position, and sudden movement acceleration, caused over 60% of false positives in a state-of-the-art AF detection algorithm. Using these findings, we designed DeepAware – a hybrid AF detection model combining deep-learning and context-aware heuristics. The DeepAware model beats the state-of-the-art on public datasets and significantly reduces the FPR on patient-operated ECG under free-living conditions. When used in a clinical setting, DeepAware can significantly reduce cardiologists' workload of manual review of FPs, allowing them to focus more on treatment than diagnostics.
In addition, this project also contributed a 259-day-long contextualized single-channel ECG arrhythmia dataset (CACHET-CADB) from patients under free-living ambulatory conditions. This database will help the broader deep learning community in building and evaluating the arrhythmia detection models that can realistically work under free-living conditions. Furthermore, it will pave the way for making the deep learning-based, end-to-end arrhythmia detection models more explainable and personalized.
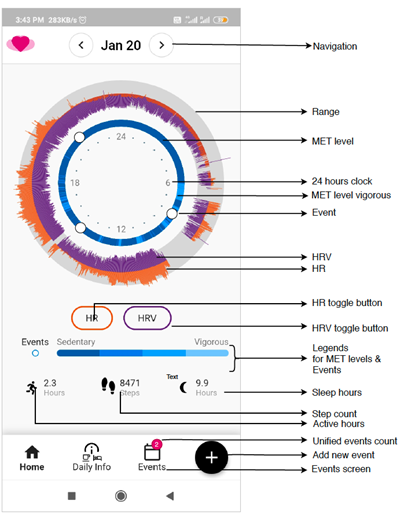 |
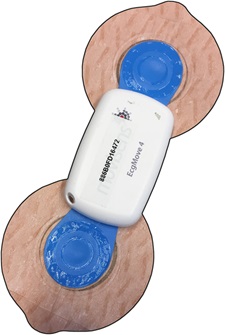
ECG Holter |
The mCardia system:
References
[1]. https://www.who.int/en/news-room/fact-sheets/detail/cardiovascular-diseases-(cvds), May 2017.
[2] https://www.ncbi.nlm.nih.gov/pmc/articles/PMC5681797
[3] https://www.ahajournals.org/doi/10.1161/circoutcomes.110.958165
[4] https://www.ncbi.nlm.nih.gov/pmc/articles/PMC5679196/
[5] https://pubmed.ncbi.nlm.nih.gov/30049358/